Matt McLure
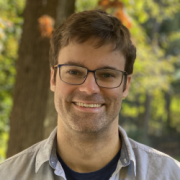
Ph.D., Computer Science, Northwestern University, 2019
M.S., Computer Science, Northwestern University, 2011
B.S., Computer Engineering, Penn State University, 2009
Dr. Matthew (Matt) McLure is a SIFT researcher specializing in Artificial Intelligence. He has published on learning by analogy, knowledge representation and reasoning, with a focus on sketched and geo-spatial data, in venues such as AAAI, CogSci and AI Magazine. Prior to joining SIFT in 2019, Dr. McLure completed his Ph.D in Artificial Intelligence from Northwestern University, where he was a member of the Qualitative Reasoning Group. His thesis work, funded by AFOSR, focused on applying machine learning techniques in feature spaces induced by sequential clustering and graph-matching across structured inputs, and a novel constraint satisfaction approach for drawing learned spatial concepts. While at Northwestern he also contributed to the Companions cognitive architecture and the open-domain sketch understanding system CogSketch.
Dr. McLure joined SIFT in 2019. He is the geospatial and network analysis lead for an IC project and a contributor to Project7, where he developed a UI for the provenance graph, an array of tools to facilitate interactive provenance analysis, and geospatial analysis capabilities. As part of DARPA’s SAIL-ON, Dr. McLure developed domain-general novelty detection methods and a hypothesis framework. He was involved in NLP work for DARPA's Habitus program, where he developed a UI for the semi-automatic curation of structured knowledge assets from authoritative documents, and recently as Knowledge Graph Learning Lead on the ARAKNI project, he applied graph-based learning techniques and ICL to detect and characterize novelties in red-force behavior.